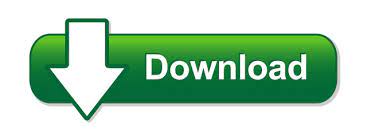
Indeed, standard models such as CBOW and fasttext are specific choices along each of these axes. Our framework is based on the well-studied problem of multi-label classification and, consequently, exposes several design choices for featurizing words and contexts, loss functions for training and score normalization. We define a new modeling framework for training word embeddings that captures this intuition. In this paper, we argue that, despite the successes of this assumption, it is incomplete: in addition to its context, orthographical or morphological aspects of words can offer clues about their meaning. Most word embeddings today are trained by optimizing a language modeling goal of scoring words in their context, modeled as a multi-class classification problem. We perform an extensive comparison of existing word and sentence representations on benchmark datasets addressing both graded and binary similarity.The best performing models outperform previous methods in both settings.īeyond Context: A New Perspective for Word Embeddings Our models are further assisted by lexical substitute annotations automatically assigned to word instances by context2vec, a neural model that relies on a bidirectional LSTM. We apply contextualized (ELMo and BERT) word and sentence embeddings to this task, and propose supervised models that leverage these representations for prediction. Usage similarity estimation addresses the semantic proximity of word instances in different contexts. Word Usage Similarity Estimation with Sentence Representations and Automatic Substitutes Furthermore, we illustrate that SURel enables us to assess optimisations of term extraction techniques when incorporating meaning shifts. We show that meaning shifts of term candidates cause errors in term extraction, and demonstrate that the SURel annotation reflects these errors. We introduce SURel, a novel dataset with human-annotated meaning shifts between general-language and domain-specific contexts. SURel: A Gold Standard for Incorporating Meaning Shifts into Term Extraction Proceedings of the Eighth Joint Conference on Lexical and Computational Semantics (*SEM 2019)Īssociation for Computational Linguistics
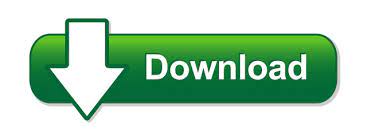